Google Cloud BigQuery is one of the most powerful data warehousing solutions available to the general public today. At Ternary, we’ve built our infinitely scalable, multi-tenant SaaS on this most-solid foundation. It was perfect for our earliest and small-scale needs, and, after three years, we still think it’s the best solution out there for companies of all sizes.
Google certainly realizes they have a winner on their hands, and recently there’s been buzz around the GCP community about pricing changes coming to better reflect how their customers use the product and the true cost to Google in serving such a powerful product to the general public. In this blog post, we’ll explore the history of BigQuery and its pricing; the value proposition of the new plans; and how companies should think about the new plans. Finally, we’ll share a case study of how we at Ternary decided to test our own strategies for BigQuery price changes.
How has BigQuery pricing changed over the years?
We can see from the Google Cloud release notes (dating all the way back to 2011!) that pricing isn’t something the BigQuery team has spent a lot of cycles on. Here’s a brief history of BigQuery pricing:
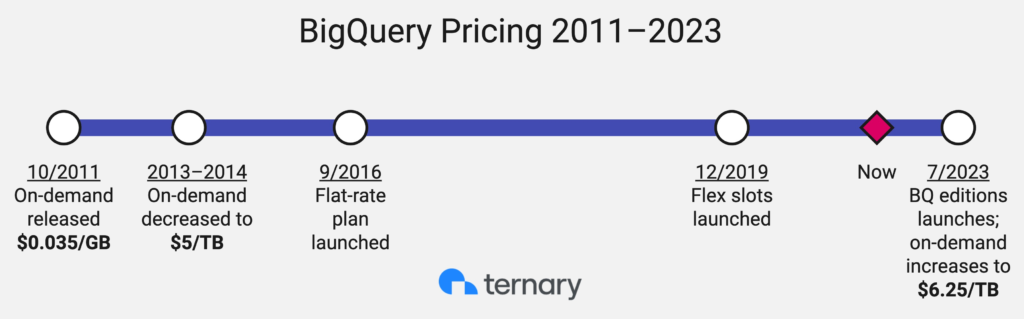
We can see that Google has generally chosen to spend its efforts on improving their querying and storage capabilities, and if they did change the pricing, they were focusing on enterprise needs (flat-rate and flex slots) rather than the needs of on-demand users. It took them five years to launch the first flat-rate plan, and three years more to revise that again, with flex slots.
Other than the absurd original pricing of $0.035/GB or $35/TB, on-demand data processing with BigQuery has cost $5/TB for most of its lifetime, and, if you consider inflation, the scheduled 25% increase simply compensates for inflation of the past 10 years, and on-demand is still very cost-effective for certain needs.
For the recent past, we can summarize the BigQuery pricing playbook for customers as follows:
- Use on-demand if you have small amounts of data to process, or process infrequently.
- Consider on-demand if your processing is very compute-intensive; on-demand processing gives you 1,000 slots of compute to use, which is not always cheap to purchase with the other plans.
- Use monthly or annual flat-rate pricing if you have consistent processing needs, or if you care more about capping costs than processing expediently.
- Use flex slots if you have bursty processing needs of large amounts of data, so long as you have automation to cancel them as soon as you’re done.
With many of these options changing or going away, these best practices must now change.
How is Ternary approaching the pricing changes?
At Ternary, prior to this pricing change, we had chosen a combination of all of these methods for our needs:
- For customers accessing their data in our product, we used on-demand, for the best performance. It’s cost-effective, because customers are not accessing and querying every single minute of the day.
- For background processing, which runs 24×7, we have used a baseline of annual flat-rate, supplemented by flex slots according to demand. We wrote our own autoscaler (which is about to become obsolete!).
Following the pricing transition in July 2023, this approach will no longer be viable. So, to prepare for transition, we researched the new options, developed hypotheses about what setups would work best for us, and ran experiments for several weeks, using different pricing models to serve our production operations. While we incurred some experimentation-related costs, these were worthy investments prior to making major new commitments (like an annual plan on a premium featureset).
At the beginning of our experimentation, we expected our production to be able to run on Standard-edition slots, based on our knowledge of them. However, when we tested them, it turned out that we were actually using some Enterprise-only features. In particular, we were making use of VPC service controls, column-level access control, and data-masking features. So, in the end, we decided to plan for a future where we would run our production on Enterprise.
The last step was to figure out what to do with our prepaid, pre-existing commitments. Per the documentation, if we did nothing, they’d all become Enterprise reservations, which is not necessarily the best option.
In the end, for the period when our old slots were still active, we decided to choose the flat-rate reservation (100% baseline slots) with a number of max slots higher than our previous baseline, to support most of the cases that we would have scaled up, and accepted lower utilization than before. Following the commitment expiration, we’ll migrate over to an autoscaling reservation to take advantage of BigQuery editions capabilities and the pay-for-what-you-use pricing model. Our experimentation helped us confirm this would be the most effective way to take advantage of our commitments.
How should your organization approach the changes?
The above example illustrates our journey through the pricing changes. But our story is somewhat unique, because we are selling a data-management and visualization product built on BigQuery. How should your organization approach them?
The answer is “It depends.” But the big takeaway we would like to share from our experience is that experimentation is crucial to find the best strategies for BigQuery price changes. To decide if BigQuery editions will be a part of your capacity-management story, we strongly recommend you start by reading about the comparison of the three editions (Standard, Enterprise and Enterprise Plus). Then, when you have an idea about what will work, experiment with the one you think is most appropriate. The good part about these pricing models is that you don’t have to be locked in to test various hypotheses.
Here are some ideas to kick-start your experimentation:
- Review your processing costs and verify that on-demand isn’t better, especially if it’s been a while since you’ve reviewed that tradeoff.
- If you were using flex slots before and don’t want to switch to on-demand, consider the autoscaling model within a BigQuery editions product.
- If you were using monthly or annual slots before, and you want to maintain cost predictability, consider the non-autoscaling model within BigQuery editions.
- Consider running certain workloads on demand, or on different editions, by segmenting the projects that you run the workloads in and the pricing model you choose for each project, for maximum optimization.
We’ve got great news if you intend to experiment: Ternary is a product that’s purpose-built for analyzing your daily BigQuery usage. While your experiments run, Ternary can help you understand the ongoing costs of your experiments, analyze the utilization of on-demand/slots that is taking place in your “labs,” and determine the best way to rightsize for your needs.
Learn more about how Ternary can help you live your best multi-cloud life.